Reza Ahmadi & Peyman Tahghighi – AGES Award
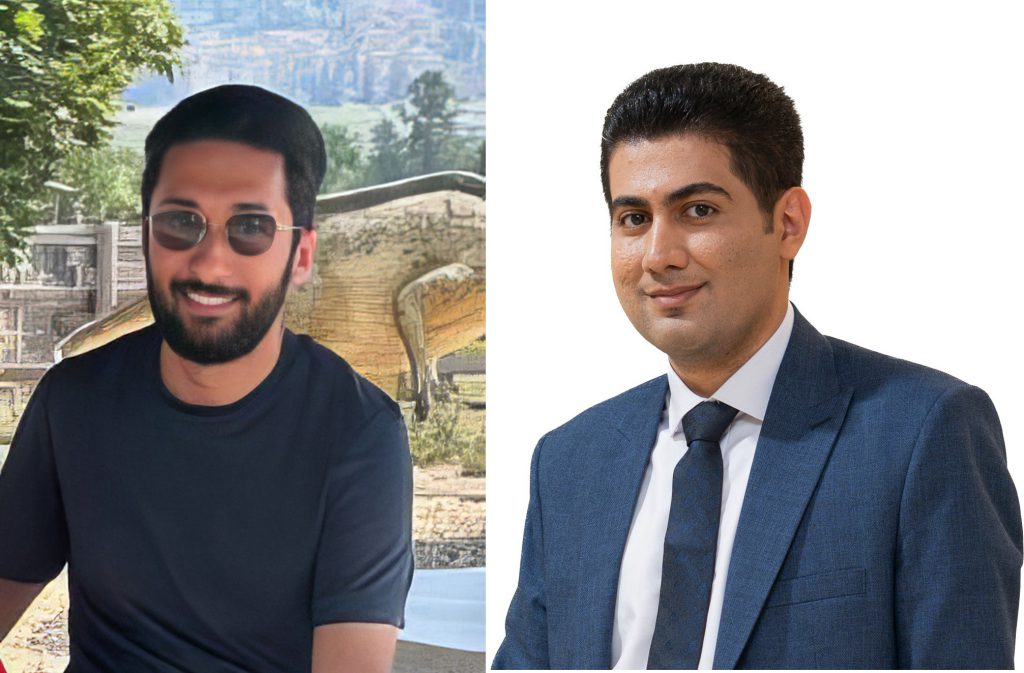
Congratulations to Reza Ahmadi (on the right) and Peyman Tahghighi (on the left), both PhD students, for receiving the Alberta Graduate Excellence Scholarship (AGES). This award recognizes their outstanding research and the significant impact it has made in their respective fields.
Predict Knee Kinematics During Stationary Cycling via Machine Learning Regression Models
To improve athletes’ performance and prevent injuries, an understanding of the kinematics of lower limbs is becoming increasingly important in rehabilitating lower extremities with cycling ergometer, particularly the hip and knee joints. Motion capture system is a common method for motion studies, however, it requires complex and expensive equipment, which is limited to the laboratory environment, might face some difficulties in finding some hidden trajectories, and are typically expensive and time-consuming. The purpose of this study is to integrate machine learning and deep learning methods with data from a motion capture system to develop a model that can predict where markers will be placed on the hips and knees on the basis of an individual’s anthropometric information and the cycling device dimensions.This study contributes significantly to biomechanical study of knee joint, offering a potential integration of predictive models and real-time monitoring of knee joint kinematics during cycling exercise with stationary ergometers. Improving and optimizing the proposed method will pave the road for developing efficient and cost-effective methods for conducting kinematics analyses. Download PDF Version
2024-04-10 Teaching and Research Excellence
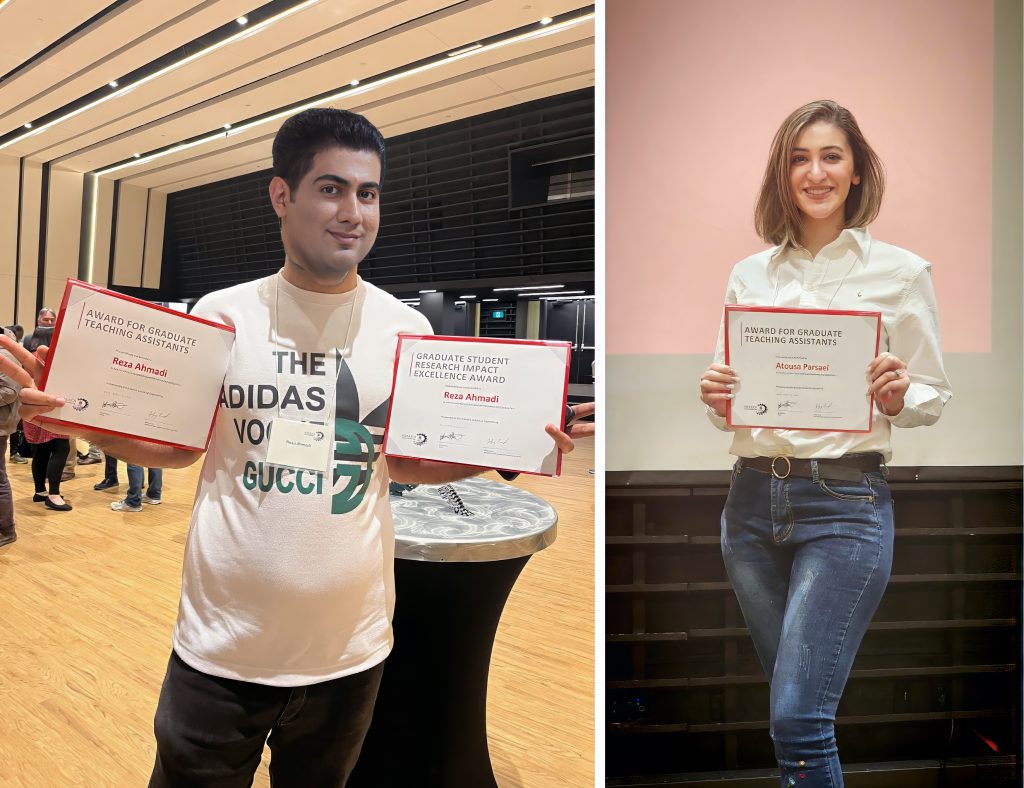
Congratulations to Atousa Parsaei and Reza Ahmadi. Reza Ahmadi and Atousa Parsaei have both been recognized with prestigious accolades from the SSE: Reza with the SSE Research Excellence Award and Teaching Assistant Award, Atousa with the SSE Teaching Assistant Award. Within iHADLab, we take immense pride in nurturing individuals who exemplify not only a commitment to groundbreaking research but also a dedication to guiding and supporting undergraduate students in their academic journeys. During the W24 semester, I had the privilege of collaborating with Reza and Atousa in delivering ENME317. Throughout this time, I witnessed their unwavering dedication in conducting labs, meticulously assessing student submissions, and contributing to various aspects of course facilitation. Their exceptional efforts did not go unnoticed, as echoed by the resoundingly positive feedback from students at the semester’s conclusion. As such, it is with great pride that we extend our heartfelt congratulations to Reza and Atousa on their well-deserved recognition. Their passion for both research and teaching serves as an inspiration to us all, and we are confident that they will continue to positively impact the academic community for years to come. On behalf of iHADLab and the department, we express our sincerest gratitude to Reza and Atousa for their invaluable contributions and unwavering commitment to excellence. PS: Reza’s body in the photo belongs to someone else (adopted with permission).
2024-04 Cortisol Biosensor Project Wins Silver Medal at Engineering Capstone
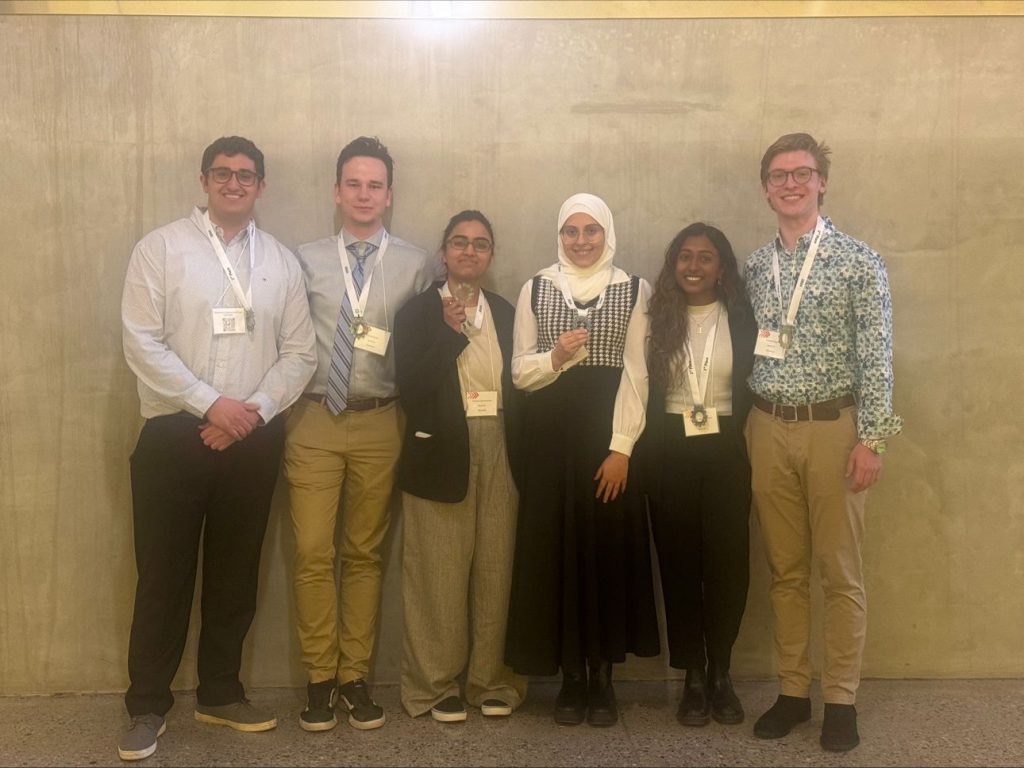
A team of engineering students from the University of Calgary’s Schulich School of Engineering, guided by their academic advisor Dr. Amin Komeili, has achieved remarkable success, securing second place at the engineering capstone fair. Their project, titled “Developing a Biosensor to Detect Cortisol Levels In The Human Body,” earned them a well-deserved silver medal in the electrical engineering department. This innovative project highlights the interdisciplinary approach to tackling real-world health monitoring challenges. Over nine months, the team, consisting of Mitchell Rhead, Ahmed Asiff, Vyshnavi Devarakonda, Carter Fuchs, and Thanusha Veeraperumal, worked diligently to develop a biosensor capable of monitoring cortisol, a key stress indicator. The development of a potentiostat circuit and a user-friendly software application for cortisol level monitoring stands out as a major achievement of this project. Acknowledgments were made to Dr. Amir Sanati Nezhad and his team for their support and contributions, which were vital to the project’s success. The project, spearheaded by students and supported by their academic and industry mentors, exemplifies the potential of collaborative engineering efforts in advancing health monitoring technologies. As the team looks forward to making further contributions in engineering, their project serves as an inspiration for future innovations in the field of health technology.
Predict Hip and Knee Kinematics During Stationary Cycling via Machine Learning Regression Models and Deep Learning Models
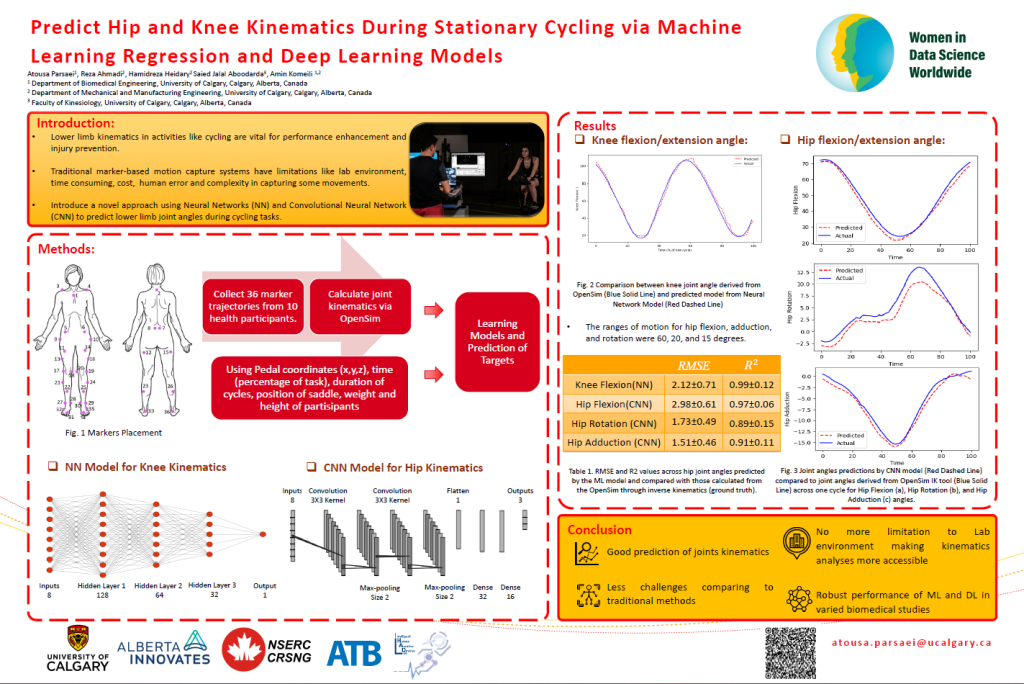
To improve athletes’ performance and prevent injuries, an understanding of the kinematics of lower limbs is becoming increasingly important in rehabilitating lower extremities with cycling ergometer, particularly the hip and knee joints. Multiple methods are being used for capturing motion and simulating kinematics of motion. Motion capture system is a common method for motion studies, which includes placement of reflective markers on the lower limbs (at about 36 locations), as well as the use of cameras to track the trajectory of markers. However, these methods require complex and expensive equipment, which is limited to the laboratory environment, might face some difficulties in finding some hidden trajectories, and are typically expensive and time-consuming. The purpose of this study is to integrate machine learning and deep learning methods with data from a motion capture system to develop a model that can predict where markers will be placed on the hips and knees on the basis of an individual’s anthropometric information and the cycling device dimensions. To address this objective, this study leveraged a Convolutional Neural Networks (CNNs) model and Neural Network (NN) regression model to predict the hip and knee joint kinematics during pedaling task on a stationary cycling ergometer. The present study involved 10 participants, comprising eight males and two females, devoid of musculoskeletal disorders, to ensure the purity and relevance of the data. The CNN model, designed with five hidden layers, was meticulously trained on this dataset. And three hidden layers were created for the NN model. Our results showed a notable accuracy in predicting hip joint kinematics parameters using CNN, with a Root Mean Square Error (RMSE) of 2.98±0.61° for hip flexion, 1.51±0.46° for hip adduction, and 1.73±0.49° for hip rotation. In addition, the R2 for the knee was 0.99±0.12 and the RMSE was 2.12±0.71. This research tried to underscore the efficacy of machine learning and deep learning in biomechanical applications, particularly for healthcare and sports applications. This study contributes significantly to biomechanical study of hip and knee joint, offering a potential integration of predictive models and real-time monitoring of hip and knee joint kinematics during cycling exercise with stationary ergometers. This technology has the potential to be employed in studies where motion capture lab is not available. Moreover, there are several advantages to this method, including the possibility of expanding it to studies of other parts of the body, and saving time and costs in comparison to capture motion systems. Improving and optimizing the proposed method will pave the road for developing efficient and cost-effective methods for conducting kinematics analyses. Download PDF Version